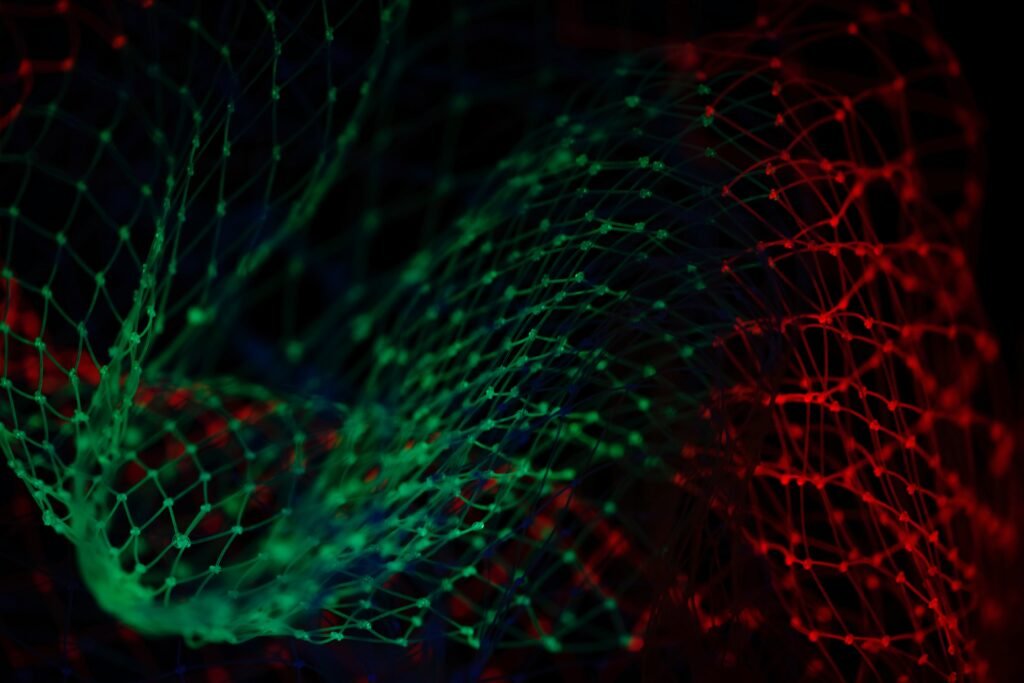
Big data is growing exponentially and businesses are turning to machine learning for actionable insights from it. Machine learning powers chatbots, language translation apps, Netflix recommendations and even self-driving cars.
However, this technology does not come without challenges. Read on to gain more insight into recent trends and advancements in machine learning.
1. Natural Language Processing
Natural Language Processing (NLP) is a branch of artificial intelligence (AI) which equips computers to understand text and voice data like humans do, for tasks like sentiment analysis, information extraction, and building chatbots.
NLP builds upon computational linguistics, using rules to detect patterns in human language. Next it incorporates statistical, machine learning and deep learning models for quick data learning without needing extensive training data sets.
Recurrent neural networks (RNNs), convolutional neural networks (CNNs), and transformer models have become key tools in NLP research, excelling at understanding sequential data to recognize relationships among words and phrases and contextualise semantics and context.
These technologies enable NLP-powered systems to identify and interpret customer queries and responses in a quick, efficient manner, reducing workload for support teams. Furthermore, NLP is used to power virtual assistants like Oracle Digital Assistant (ODA), Siri, Cortana and Alexa that help customers by answering their inquiries or providing the most pertinent information.
2. Deep Learning
Machine learning (ML) allows computers to learn from data and make decisions without being explicitly programmed, which has wide-ranging applications from detecting credit card fraud and automatically tagging Facebook friends, to predicting sales trends and more.
Yann LeCun and Yoshua Bengio’s breakthrough in machine learning came through their paper on “Deep Learning. Deep Learning utilizes computational models with multiple processing layers that learn representations of data with increasing levels of abstraction – also referred to as feature learning.
Deep learning programs differ from traditional machine learning in that they learn by example rather than human programmer input, similar to toddlers learning about what a dog looks like by pointing and saying “dog” or “not a dog”. This method provides for automated feature engineering while simultaneously helping ML algorithms handle unstructured data, which becomes increasingly diverse as data sets expand and diversify. Furthermore, ML algorithms can identify complex relationships among features in data that help increase accuracy.
3. Quantum Computing
Quantum computing could revolutionize machine learning and artificial intelligence (AI) models – something which has already started happening!
Quantum computers can handle massively complex data sets that conventional computers cannot. This technology could be utilized for tasks like classifying large amounts of images or data sets quickly while speeding up mathematical algorithms.
Technology can also enable faster and more powerful machines by optimizing algorithms that power computer hardware. For instance, using quantum algorithms designed specifically for machine learning to increase natural language processing accuracy – crucial in applications like chatbots or virtual assistants.
Though organizations may take five to 10 years before they use quantum computing meaningfully, it’s worth keeping up with its development. Data centers in particular should partner with quantum computing players and hire skilled talent as part of their preparation plan for quantum computing’s implementation; meantime they should invest in digital transformation efforts while expanding their data sets.
4. Self-driving cars
Machine learning (ML) algorithms have become an essential element in autonomous vehicle technology, allowing cars to recognize objects around them and decide the most efficient path between points A and B. To accomplish this feat, their software processes sensor input from cameras, sensors, LiDAR (light detection and ranging, similar to radar) and radar in order to identify all relevant sources in its environment.
Autonomous vehicles must communicate with traffic infrastructure to avoid collisions and maintain smooth operations, and communicate in real-time with other drivers to improve passenger comfort and reduce accident risks. Machine learning technology enables these cars to exchange data with drivers in real-time for better safety, reduce risks of accidents, detect security breaches via anomaly detection systems such as machine learning systems that identify anomalous behaviour, protect privacy and secure both passengers and vehicles in a manner which helps protect both privacy and security simultaneously. While self-driving car technology appears promising at present – its capabilities still need further development! This future looks very bright indeed! But its technology remains very much under development compared with what will come later down the track compared with what might become available once self-driving car technology takes off completely! But as technology has yet to reach maturity so it must remain cautious.
5. Machine learning in transportation
Machine learning is revolutionizing our lives in many ways, from product recommendations for customers to cybersecurity breaches and self-driving cars. Thanks to new technologies that gather and analyze large amounts of data quickly, the potential uses for machine learning is seemingly limitless.
Machine learning will not only facilitate automation but will also transform healthcare by providing personalized treatments and diagnoses. Furthermore, machine learning will assist smart cities by optimizing resource management while spurring scientific breakthroughs through rapid data analysis.
Transport industries are making use of AI in numerous ways, from optimizing logistics planning and improving traffic congestion to self-driving cars and intelligent public transit services. Airlines are using AI to predict weather conditions and improve ETA accuracy.